Overcoming some uncomfortable truths about data
Share
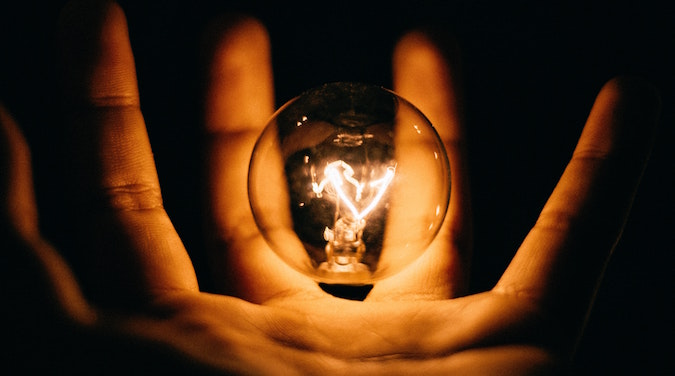
Cécile Ferré explains why the quality of data-led insights is dependent on not only the metrics you choose but having a holistic and strategic approach to your data-sets.
I recently attended a data analytics workshop, which was facilitated by a professional and expert in the field. As he proceeded to describe a typical day in a data analyst’s life, he unexpectedly made a candid comment on how “unsexy” the job was for the most part.
As a data analyst, he explained, one would spend the vast majority of their time on the more mundane tasks of preparing the data for analysis, with only a small portion spent on the more intellectually-rewarding (or so was implied) tasks of analysing and interpreting said data, or presenting insights and recommendations.
Cognilytica, an IA specialist consultancy, further confirms this by stating that data wrangling (as data preparation is otherwise known) of various sorts takes up about 80 percent of the time consumed in a typical AI project.
This has also been my experience working on various data projects for Australian brands. Yet, data wrangling is also one of the critical pieces of the data analysis puzzle.
Fundamentally, as a business, the quality of your data-led insights is as good as the quality of the data you work with or ‘feed the AI machine’. Yet one of the biggest hurdles faced by businesses today is data quality – or lack of. This makes data wrangling (i.e. the process of selecting, extracting, importing, cleaning and mapping the data into a fit-for-analysis format), together with data interpretation, an essential component of your business’s data analysis workflow and one that ultimately impacts the reliability of your findings.
More to the point, the quality of your insights depends not only on choosing the right metrics and data points to focus on (be it to measure performance, help solve a business problem or identify a market opportunity), but also on how you clean, structure, visualise and interpret them. And whilst using AI techniques and BI tools (such as NLP or data visualisation software) greatly assists this process, it doesn’t stop data, human or AI-related issues from creeping in and potentially ‘tainting’ your analysis and findings, with some issues more common than others.
Chief among them, and perhaps the most controversial, is the bias found in both humans and AI systems. Indeed, much ink has been spilled on historical data where groups are underrepresented or discriminated against, and how it can create bias in algorithms. Apple experienced this issue when its new credit card algorithm was accused of gender discrimination.
Even when every effort is made to remove bias and neutralise data sets, AI-generated data outputs can’t be fully trusted. Algorithms often require you to detect, correct or remove unneeded, inaccurate or corrupt information. For this reason, knowingly, some social media analytics platforms let you override sentiment classifications at post or topic level, when their sentiment scoring algorithm fails to read the context correctly. This is also why, on a recent project, we had to cleanse the data pulled from a search analytics platform, whose algorithm was failing to recognise branded terms in live search queries and incorrectly classifying them as unbranded (for example, ‘commonwealth bank’ was one such term).
Critically also, the risk of human bias is present every step of the way. As a data analyst, you always start with one or more hypotheses that you are trying to validate or disprove, and it is very tempting to select data that will say what you want it to say. In her book Made by Humans: the AI Condition, leading data expert Ellen Broad nicely sums it up: “Before we know it, we’re just subconsciously selecting and relying on the data points that fit our pre-existing hypothesis (…) How we interpret data – the data we think is relevant and what we think it tells us – is informed by our own experiences and prejudices.”
Additionally, the data you need may not exist, as was the case for Amazon’s “Go” stores. The retail giant had to create large volumes of ‘training data’ (in the form of videos of both real and virtual shoppers browsing shelves, picking up or returning items to shelves, etc.) to feed the AI system used to operate the stores.
So, what mitigation strategies should one consider to safely navigate the data minefield?
As a business, there are ways you can mitigate these and other deficiencies you may experience along the way, and below we explore a few.
First, the make-up of your data and insights team should be carefully considered. From experience, data analysts and data scientists working in close collaboration with business and data strategists as the ‘storytellers’ tend to deliver the best outcomes. Strategists are indeed uniquely placed to elevate the detail that matters, by connecting the dots and aligning the key findings to the business goal or priority in focus. A composite team with different skills, perspectives and abilities (left-brained vs right-brained) will also generate a healthy amount of debate around both limitations and insights, helping solve issues and minimize biases through group discussions.
Equally key is the team’s aptitude for dealing with uncertainty or ambiguity around data, as well as its ability to find quick workarounds when difficulties arise. Data modelling and analysis are typically highly iterative, with no guaranteed results. It all starts with a hunch or an assumption, which may or may not be realised, and ends with observations that could be interpreted in a number of ways. And though they can’t be eradicated entirely, uncertainty and ambiguity may be mitigated to an extent through hiring talent with domain or industry knowledge. Years of experience working in a particular vertical will indeed help validate data patterns and trends, or detect inconsistencies.
Methodology matters too – in the way your team approaches a particular data brief or project. In some instances, it may be best to start with a small data set and in others, with a broad query that generates high volumes for your team to refine and iterate on. For example, the latter is recommended for exploratory listening queries; unnecessary ‘noise’ gets removed iteratively as you surface themes of conversation and narrow your analysis down to those that matter around a particular product, brand or category.
Finally, sometimes more data means more accuracy. Where possible and relevant, it may be advisable to validate your findings by cross-referencing multiple data sets to paint a more accurate picture. For example, one way of mitigating ‘bragging’ that may surface through your social media listening around a particular topic is to cross-reference these online behaviours with offline behaviours from another reputable source (such as Roy Morgan).
All things considered…
Data quality is critically important and yet it is hardly ever reached. The data analysis process is rife with imperfections and limitations, from data preparation through to interpretation. Compromises and judgment calls have to be made along the way, impacting the reliability of your learnings and recommendations – favourably or not. However, risks can be mitigated and issues overcome not only with the right data infrastructure, mining tools and methodology in place, but also with talent by your side with the right mix of expertise, resourcefulness and a special flair for dealing with the uncertainty and ambiguity that prevail. And, although a 100pc data accuracy or completeness may never be attained, your data-led outputs and findings should be accurate or complete enough to support your business decision-making with confidence.
Cécile Ferré is strategy partner of insights, data & analytics at Saatchi & Saatchi Australia.
Photo by Rohan Makhecha on Unsplash.